Four published contributions, in October 2023, in the IEEE International Conference on Biomedical Circuits and Systems (BioCAS) from CenBRAIN Neurotech. Congratulations to PhD students: Di Wu, Jinbo Chen, Wenjun Zou, and Junzhe Wang. IEEE BioCAS is a premier international forum for presenting interdisciplinary research and development activities at the crossroads of medicine, life sciences, physical sciences, and engineering that shape tomorrow's medical devices and healthcare systems. Chair Prof. Mohamad Sawan, the founding director of CenBRAIN Neurotech, led two PhD students (Junzhe Wang and Jinbo Chen) to actively participate in this conference in Toronto, Canada. This marked a significant milestone, as it was the first time that our students had the opportunity to engage in-person in an international conference after the COVID-19 pandemic. Junzhe Wang delivered an oral presentation, while Jinbo Chen showcased his research in the poster session.
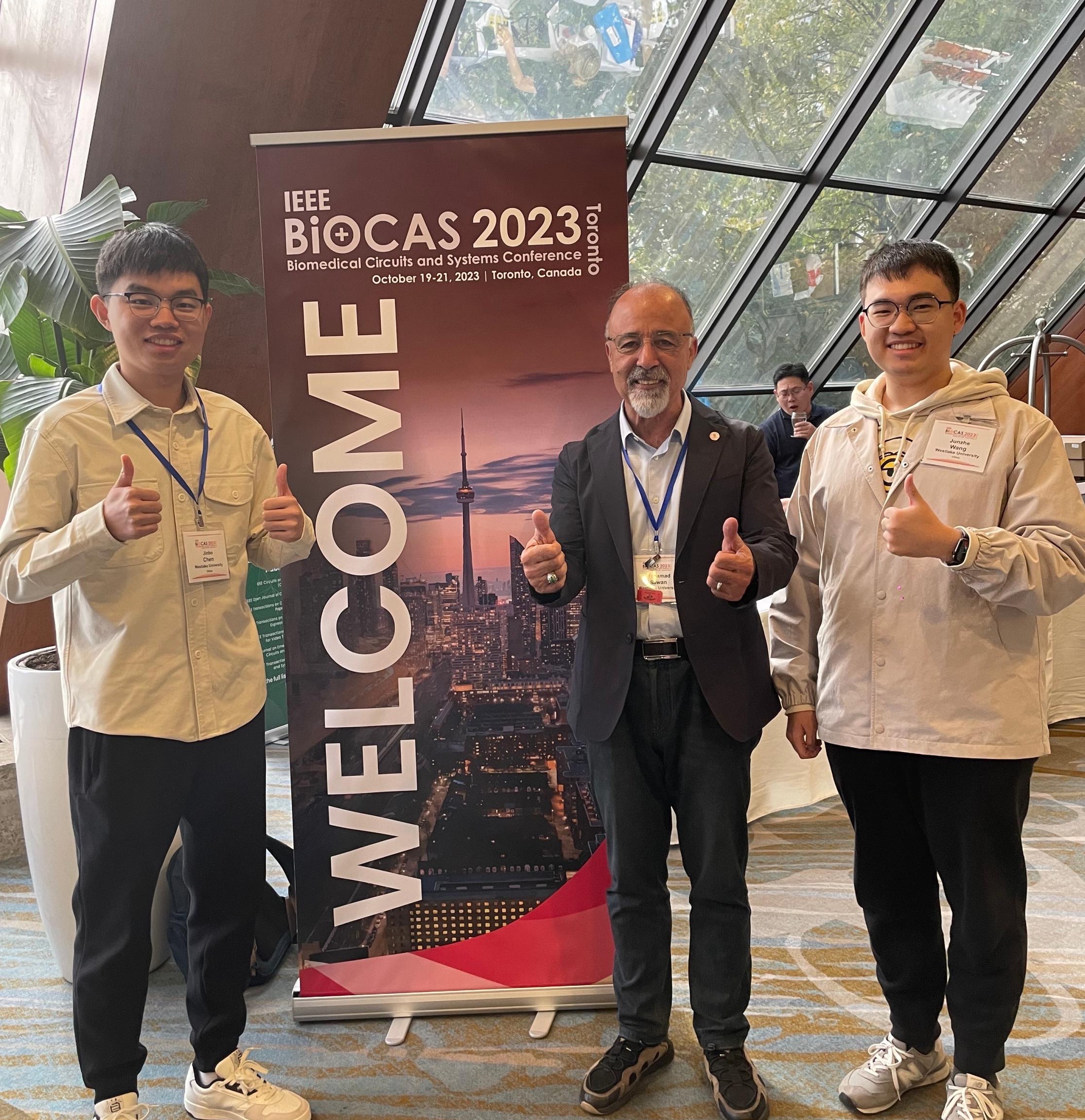
Junzhe Wang conveyed his honor in representing his university at BioCAS in Toronto, where he expanded his horizons through diverse interactions and insightful lectures. He remarked, "This participation is a valuable experience that has greatly benefited me." Jinbo presented his research advancements in bionic spike detectors at BioCAS, sparking discussions with global researchers. He expressed, "Participating in this year's BioCAS was a wonderful and unforgettable experience. I hope to actively pursue more participation opportunities in the future!"
Paper #1
J Wang, et al., “An Event-driven Neural Signal Processor for Closed-loop Seizure Prediction”, IEEE Biomedical Circuits and Systems Conference (BioCAS), October 2023.
https://epapers2.org/biocas2023/ESR/paper_details.php?paper_id=5320
Abstract
Epileptic seizure prediction which enables timely intervention, is critical for closed-loop seizure controls. However, real-time neural signal processing, one of the effective approaches to address seizure prediction, poses a significant challenge due to its high power consumption, especially for implantable medical devices. In this paper, we propose a neural signal processor featuring an event-driven processing manner, designed for low-power, high-precision real-time epileptic seizure prediction. Our system monitors neural signals using a binary neural network (BNN) to detect any possible abnormal events and reconfigures to a convolutional neural network (CNN) for high-precision prediction and to reject false alarms. Validated with real-world neural signals from patients with epilepsy, our system demonstrates an F1 score of 0.97 in predicting seizures, outperforming existing state-of-the-art approaches. Thanks to the event-driven design, the average power consumption of our system is less than 200 µW, making it suitable for implantable closed-loop medical devices.
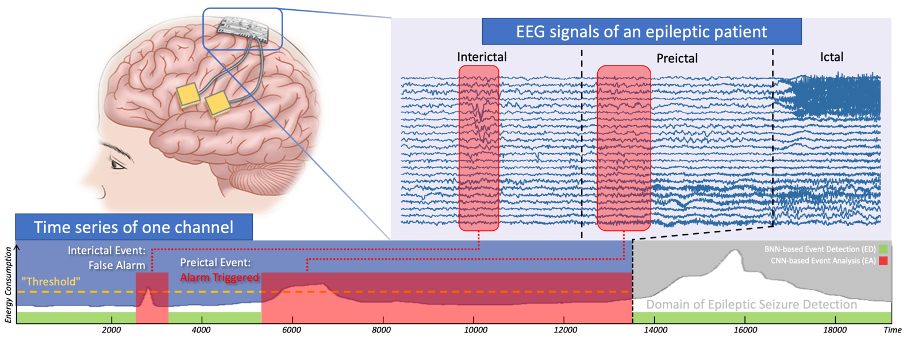
Fig.1: The event-driven design in our neural signal processor. For epileptic seizure prediction (ESP) tasks, a binary neural network (BNN) is used to host a long-term event detection (ED). If it detects any event, the processor is switched to a CNN-based event analysis (EA) mode with higher precision, to accurately check whether a full seizure is about to take place.
Paper #2
W. Zou, R. Eskandari, X. Liu, J. Chen, J. Yang and M. Sawan, “Wireless Data Transceivers for Brain-Machine Interfaces”, IEEE Biomedical Circuits and Systems Conference (BioCAS), October 2023.
https://epapers2.org/biocas2023/ESR/paper_details.php?paper_id=5322
Abstract
By acquiring and transmitting the human brain’s neural activities, brain-machine interface (BMI) technology enables direct communication between the brain and an external device. This technology opens up new ways to investigate brain function and treat neurological disorders. However, the increasing demands for large-scale neural recording impose a challenge for the wireless telemetry module, requiring it to provide a high data rate within a restricted power budget. In this paper, We present a comparative analysis of commonly adopted wireless data transmission technologies for BMI systems. The advantages and limitations of different technologies including Bluetooth, Zigbee, Wi-Fi, WMTC, MICS, and ultra-wideband (UWB) are identified, compared, and analyzed in a quantitative manner. Furthermore, solutions to build future wireless transmission technologies for high-throughput and low-power implantable BMIs are projected.
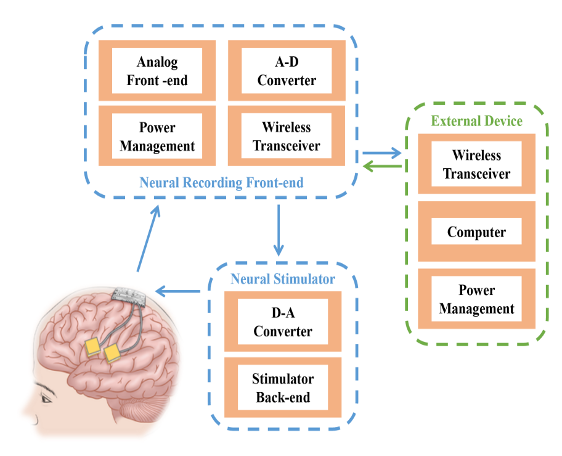
Fig. 1: Schematic diagram of a BMI framework.
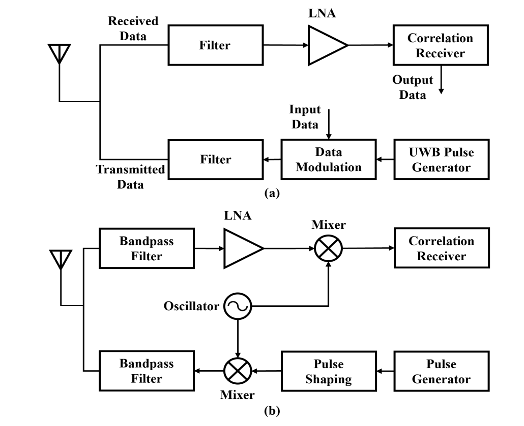
Fig.2: UWB Transceivers architecture: (a) Carrier-less based systems (b) Carrier-based devices
Paper #3
J. Chen, X. Liu, H. Wu, F. Tian, W. Zou, M. Katebi, R. Eskandari, J. Yang, M. Sawan, “A Clockless Robust Bionic Spike Detector for Implantable Brain-Computer Interfaces”, IEEE Biomedical Circuits and Systems Conference (BioCAS), October 2023.
https://epapers2.org/biocas2023/ESR/paper_details.php?paper_id=5325
Abstract
The application of Brain-Computer Interfaces (BCIs) in neuroscience and neurological disorders has witnessed extensive adoption. With the shift towards implantable wireless BCIs featuring higher channel counts, the surge in data volume presents challenges in bandwidth and transmission power. On-implant feature extraction and spike detection are crucial to reduce data bandwidth, but suffers from limited power budgets. Optimizing power consumption while maintaining detection accuracy are thus vital for efficient BCI systems. In this paper, we present a clockless robust bionic low-power spike detector for implantable BCI. The proposed spike detector achieves low-power and event-driven clockless bionic detection by inter-module co-design that integrates pipelined analog-to-spike conversion sampling, neural signal feature extraction, and 2-stage depolarization and repolarization based spike detection. Moreover, a sampling-aware unsupervised firing-rate-based detection threshold search method is proposed to achieve robust and adaptive detection. Simulation results demonstrate that the proposed spike detector achieves 90.9% - 100% average detection accuracy on a commonly used synthetic dataset with only 0.95 uW power consumption per channel.
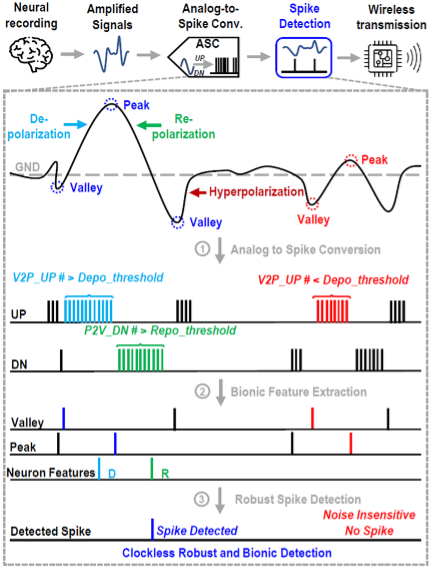
Fig. 1: Overview of the proposed clockless robust bionic spike detector for implantable BCI.
Paper #4
D. Wu, J. Yang, and M. Sawan, “A Task-aware Compression for Wearable Sensor Data”, IEEE Biomedical Circuits and Systems Conference (BioCAS), October 2023.
Abstract
The progress made in the field of neuroscience, along with the advancement of deep learning (DL), has led to notable achievements with wearable biomedical sensors. However, the large amount of data generated by wearable sensors poses a significant challenge for data transmission due to the limited resource capacity of wearable and implantable devices. We present in this paper presents a task-aware signal compression framework, dubbed task-aware compression (TAC), for wearable sensor data that considers the specific task being performed and the data reconstruction process. The proposed algorithm uses a learnable compression matrix that is trained by a neural network to extract task-related features to reduce the dimension of the data. The compressed data is utilized for task-related analysis and high-fidelity reconstruction. The proposed methodology is evaluated with the task of seizure prediction using electroencephalogram (EEG) data, and experimental results illustrate that with a compression ratio of 1/16, the method is able to reduce maximum transmission and data analysis related power by more than 90 %, and the prediction accuracy degradation is below 3 %.
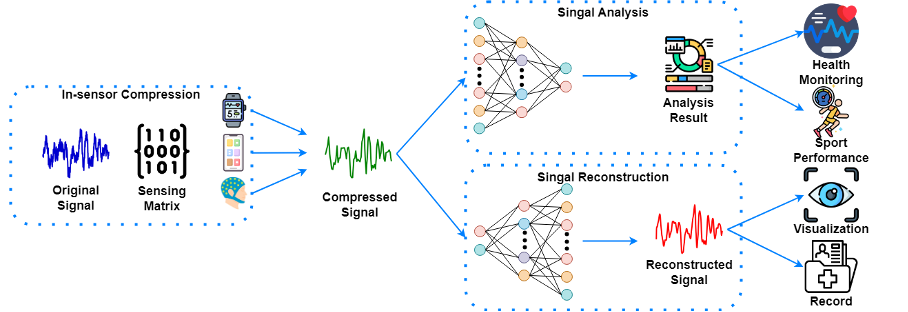
Fig. 1: Overview of the proposed TAC.