Titled “A 0.99-to-4.38 uJ/class Event-Driven Hybrid Neural Network Processor for Full-Spectrum Neural Signal Analyses”, this contribution has been accepted in IEEE Transactions on Biomedical Circuits and Systems, where we demonstrated a general-purpose physiological signal processor which combines the hybrid neural network, event-driven, and reconfigurable design paradigms.
Congratulations to Shiqi Zhao and to this paper’s co-authors (Jie Yang, Junzhe Wang, Chaoming Fang, Tengjun Liu, Shaomin Zhang, Mohamad Sawan) for this excellent achievement.
Reference
S. Zhao, J. Yang, J. Wang, C. Fang, T. Liu, S. Zhang, M. Sawan. A 0.99-to-4.38 uJ/class Event-Driven Hybrid Neural Network Processor for Full-Spectrum Neural Signal Analyses[J]. IEEE Transactions on Biomedical Circuits and Systems, 2023. DOI: 10.1109/TBCAS.2023.3268502.
Abstract
Versatile and energy-efficient neural signal processors are in high demand in brain-machine interfaces and closed-loop neuromodulation applications. In this paper, we propose an energy-efficient processor for neural signal analyses. The proposed processor utilizes three key techniques to efficiently improve versatility and energy efficiency. 1) Hybrid neural network design: The processor supports artificial neural network (ANN)- and spiking neural network (SNN)-based neuromorphic processing where ANN is used to support the processing of ExG signals and SNN is used for handling neural spike signals. 2) Event-driven processing: The processor can perform always-on binary neural network (BNN)-based event detection with low-energy consumption, and it only switches to the high-accuracy convolutional neural network (CNN)-based recognition mode when events are detected. 3) Reconfigurable architecture: By exploiting the computational similarity of different neural networks, the processor supports critical BNN, CNN, and SNN operations with the same processing elements, achieving significant area reduction and energy efficiency improvement over those of a naive implementation. It achieves 90.05% accuracy and 4.38 uJ/class in a center-out reaching task with an SNN and 99.4% sensitivity, 98.6% specificity, and 1.93 uJ/class in an EEG-based seizure prediction task with dual neural network-based event-driven processing. Moreover, it achieves a classification accuracy of 99.92%, 99.38%, and 86.39% and energy consumption of 1.73, 0.99, and 1.31 uJ/class for EEG-based epileptic seizure detection, ECG-based arrhythmia detection, and EMG-based gesture recognition, respectively.
More information can be found at the following link:
https://ieeexplore.ieee.org/document/10105432
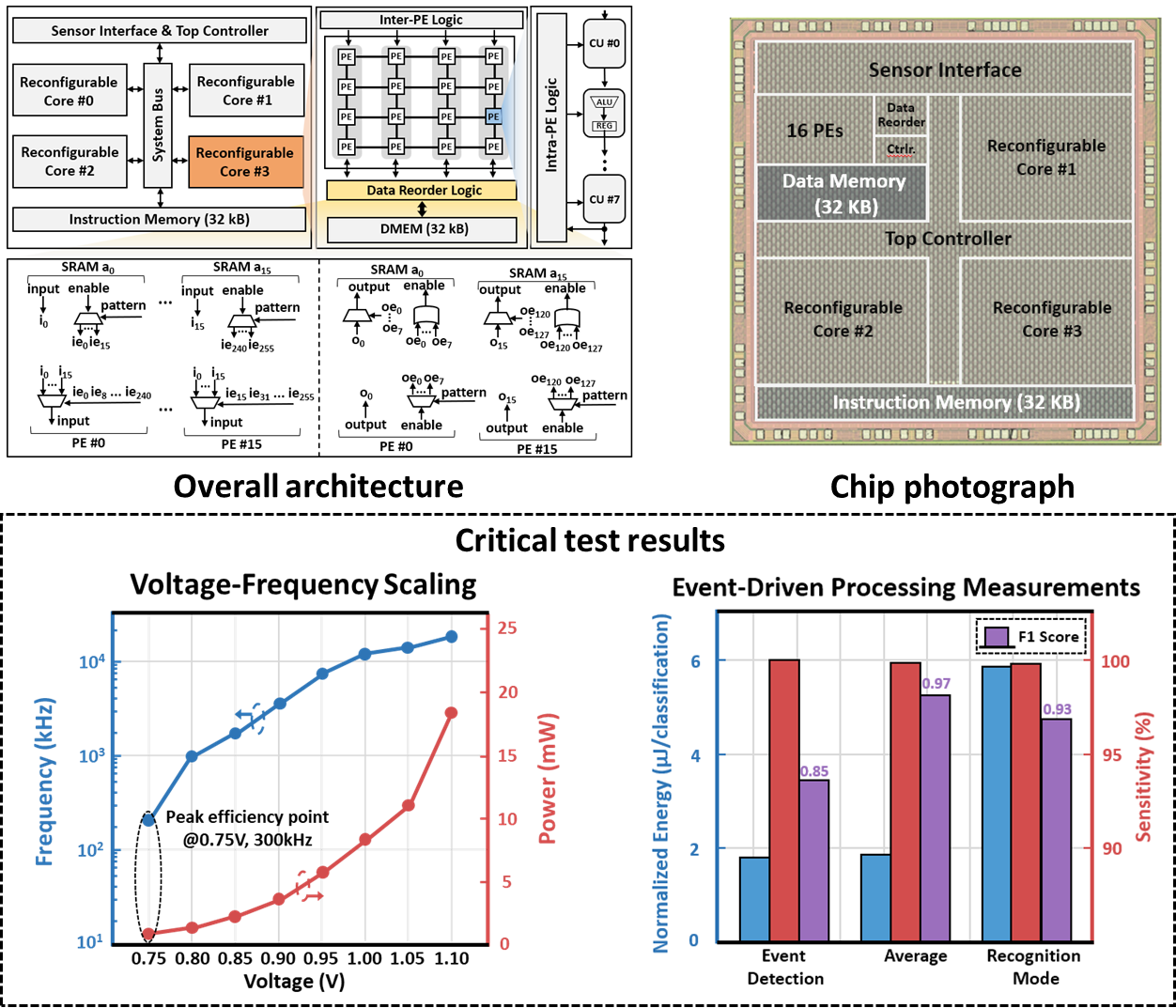
The figure shows the overall architecture, chip photograph, and critical test results of the proposed processor. The test results indicate that the proposed processor operates within a normal working voltage range of 0.75V to 1.1V and a frequency range of 300kHz to 20MHz. The lowest power consumption of 142.9μW and peak energy efficiency of 0.99μJ were achieved at the voltage of 0.75V and the frequency of 300kHz. In addition, the proposed processor achieves single-inference energy consumption at the μJ level.
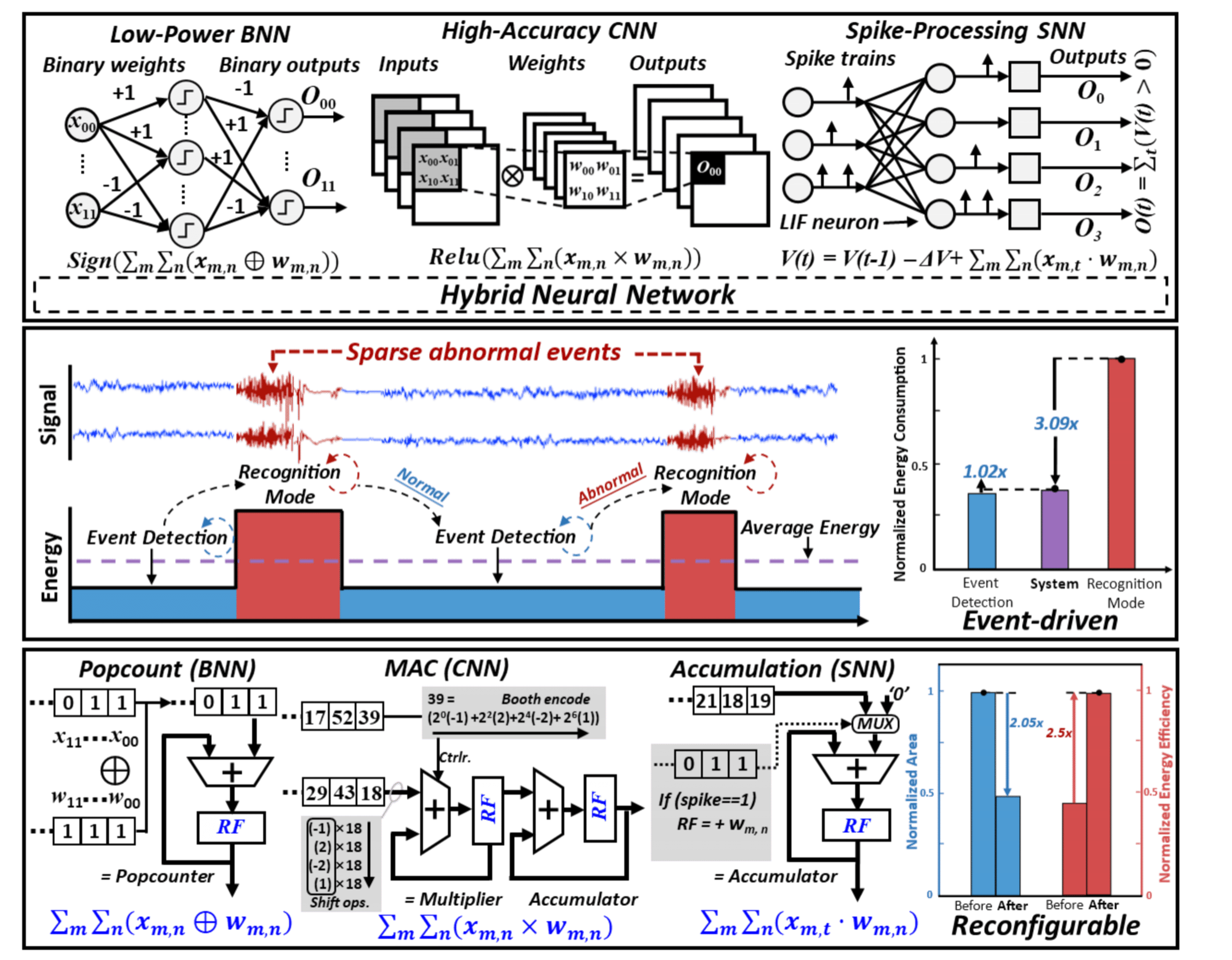
The figure shows three main features of the proposed neural signal processor: 1) hybrid neural network design; 2) novel event-driven design; and 3) reconfigurable chip architecture and circuit design.