Titled “Software-Hardware Co-Design for Energy-Efficient Continuous HealthMonitoring Via Task-Aware Compression”, this contribution has been published in the IEEE Transactions on Biomedical Circuits and Systems. In this publication, we introduced a health monitoring framework that performs task-aware signal compression to preserve task-relevant information on sensor with a low computation cost. Congratulations to Di Wu and to this paper’s co-authors (Shiqi Zhao, Jie Yang, and Mohamad Sawan) for this excellent achievement.
Reference
D. Wu, S. Zhao, J. Yang and M. Sawan, "Software-Hardware Co-Design for Energy-Efficient Continuous HealthMonitoring Via Task-Aware Compression," in IEEE Transactions on Biomedical Circuits and Systems, doi: 10.1109/TBCAS.2023.3238719.
Abstract
Low power consumption associated with data transmission and processing of wearable/implantable devices is crucial to ensure the usability of continuous health monitoring systems. In this paper, we propose a novel health monitoring framework where the signal acquired is compressed in a task-aware manner to preserve task-relevant information at the sensor end with a low computation cost. The resulting compressed signals can be transmitted with significantly lower bandwidth, analyzed directly without a dedicated reconstruction process, or reconstructed with high fidelity. Also, we propose a dedicated hardware architecture with sparse Booth encoding multiplication and the 1-D convolution pipeline for the task-aware compression and the analysis modules, respectively. Extensive experiments show that the proposed framework is accurate, with a seizure prediction accuracy of 89.70 % under a signal compression ratio of 1/16. The hardware architecture is implemented on an Alveo U250 FPGA board, achieving a power of 0.207 W at a clock frequency of 100 MHz.
More information can be found at the following link:
10.1109/TBCAS.2023.3238719
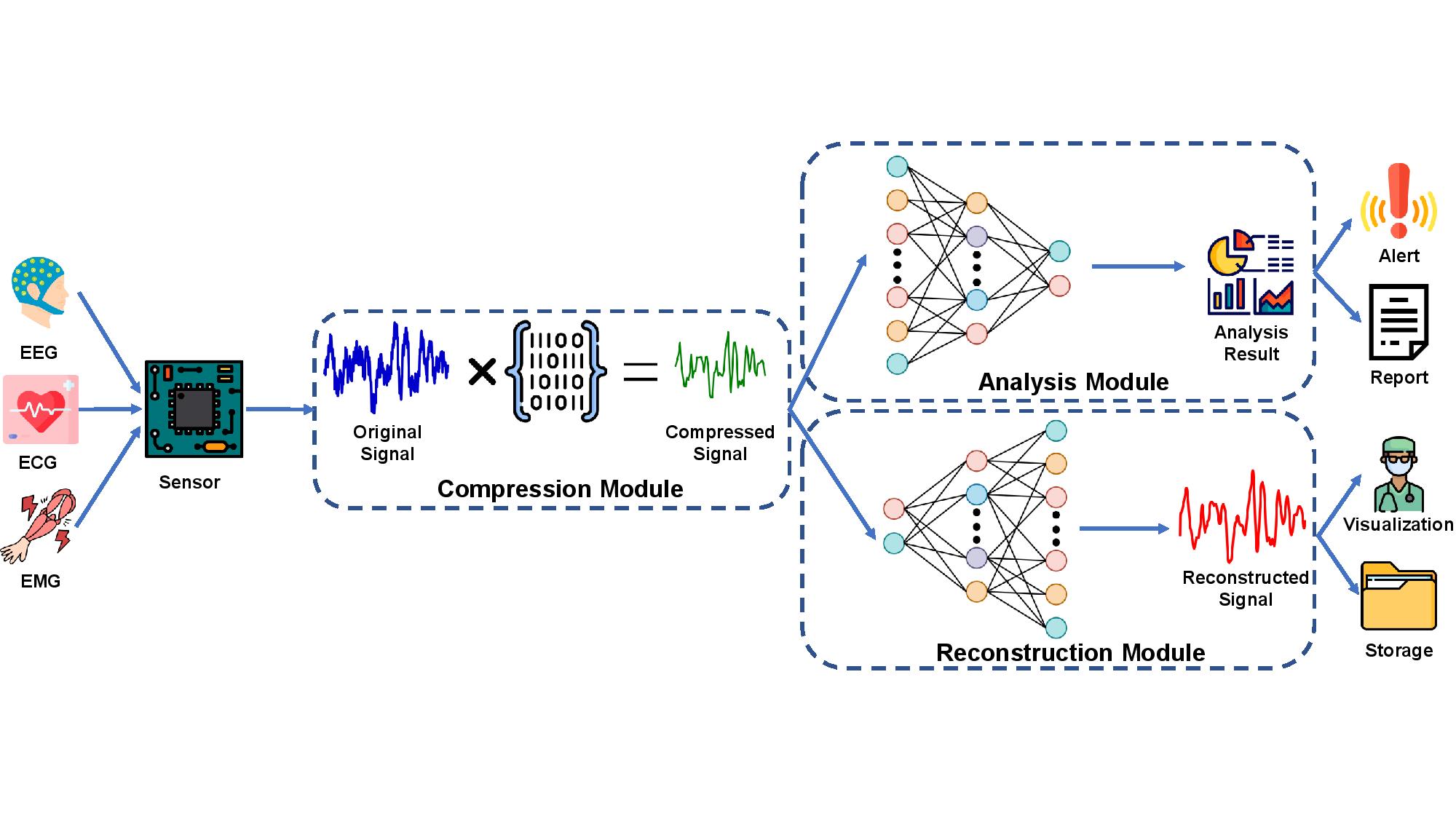
A conceptual illustration of the training process of the proposed CHM framework.