Titled “NeuroSEE: A Neuromorphic Energy-efficient Processing Framework for Visual Prostheses”, this contribution has been published in the IEEE Journal of Biomedical and Health Informatics. In this publication, we propose a data processing framework that combines a spike representation encoding technique and a bio-inspired processing method. The proposed NeuroSEE predicts the response of the primary visual cortex in an energy efficient manner, making it a powerful tool for visual prostheses. This contribution was featured on cover page (See below). Congratulations to Ph.D. student Chuanqing Wang and to this paper’s co-authors for this excellent achievement.
Citation
C. Wang, J. Yang and M. Sawan, "NeuroSEE: A Neuromorphic Energy-Efficient Processing Framework for Visual Prostheses," in IEEE Journal of Biomedical and Health Informatics, vol. 26, no. 8, pp. 4132-4141, Aug. 2022, doi: 10.1109/JBHI.2022.3172306.
Abstract
Visual prostheses with both comprehensive visual signal processing capability and energy efficiency are becoming increasingly demanded in the age of intelligent personal healthcare, particularly with the rise of wearable and implantable devices. To address this trend, we propose NeuroSEE, a neuromorphic energy-efficient processing framework that combines a spike representation encoding technique and a bio-inspired processing method. This framework first utilizes sparse spike trains to represent visual information, and then a bio-inspired spiking neural network (SNN) is adopted to process the spike trains. The SNN model makes use of an IF neuron with multiple spike firing rates to decrease the energy consumption without compensating for prediction performance. The experimental results indicate that when predicting the response of the primary visual cortex, the framework achieves a state-of-the-art Pearson correlation coefficient performance. Spikebased recording and processing methods simplify the storage and transmission of redundant scene information and complex calculation processes. It could reduce power consumption by 15 times compared with the existing Convolutional neural network (CNN) processing framework. The proposed NeuroSEE framework predicts the response of the primary visual cortex in an energy efficient manner, making it a powerful tool for visual prostheses.
More information can be found at the following link:
https://ieeexplore.ieee.org/abstract/document/9767670
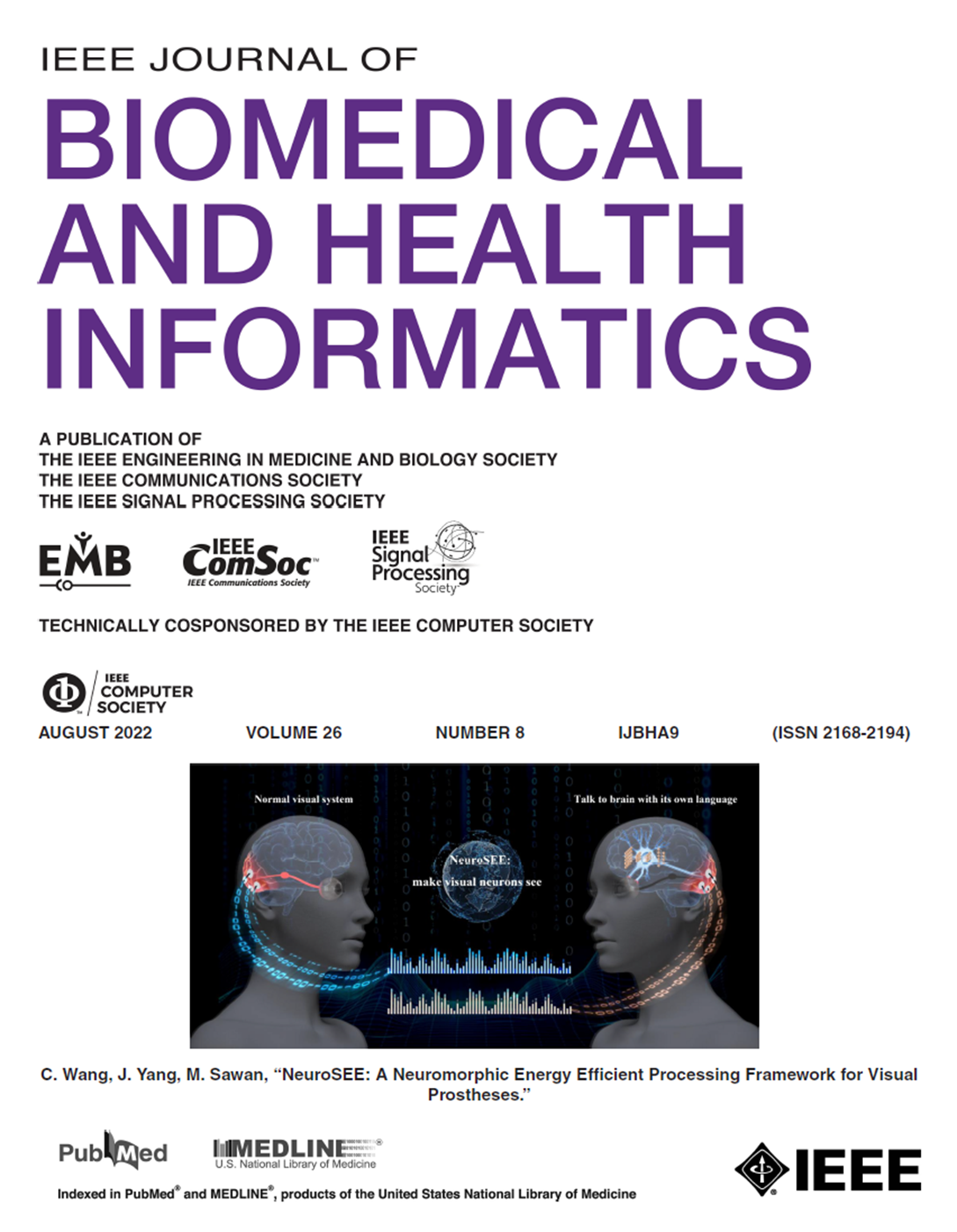