Deep learning associated with neurological signals is poised to drive major advancements in diverse fields such as medical diagnostics, neurorehabilitation, and brain-computer interfaces. The challenge in harnessing the full potential of these signals lies in the dependency on extensive, high-quality annotated data, which is often scarce and expensive to acquire, requiring specialized infrastructure and domain expertise.
To address these challenges, CenBRAIN Neurotech Center of Excellence recently published a study on the IEEE Journal of Biomedical and Health Informatics journal. We propose Neuro-BERT, a novel self-supervised framework tailored for neurological signals. Neuro-BERT works reasonably well without any augmentations, thereby avoiding any potential corruption of the original data. Additionally, this simplifies the model structure while maintaining robust performance, making it particularly suitable for practical applications in neurological research.
Congratulations to our senior PhD student Di Wu and to co-authors (Jie Yang and Mohamad Sawan) for this achievement.
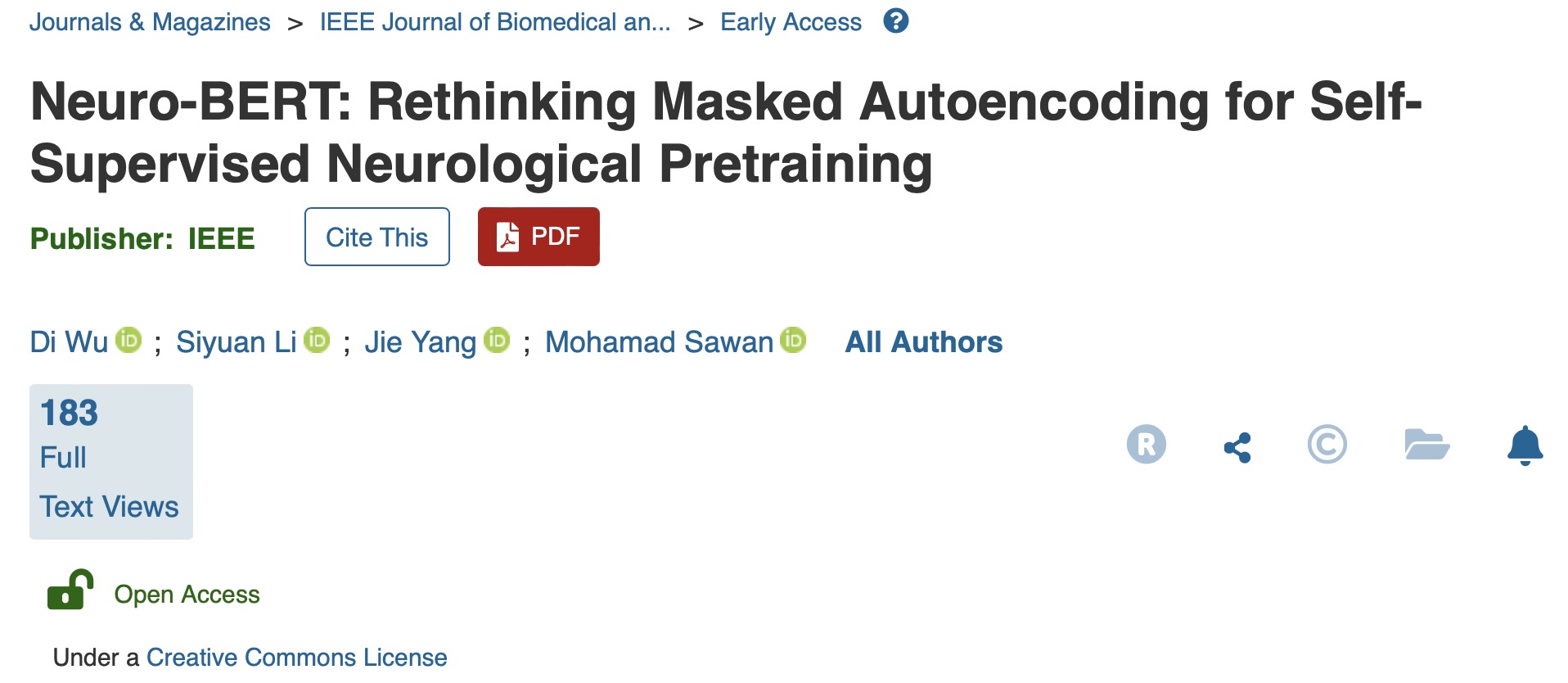
Reference
WU D., LI S., YANG J., SAWAN M., “Neuro-BERT: Rethinking Masked Autoencoding for Self-Supervised Neurological Pretraining”,IEEE Journal of Biomedical and Health Informatics, Online, 2024.
https://ieeexplore.ieee.org/document/10561479
Abstract
Frequency and phase distribution of neurological signals can reveal intricate neurological activities. We propose a novel pre-training task dubbed Fourier Inversion Prediction (FIP), which randomly masks out a portion of the input signal and then predicts the missing information using the Fourier inversion theorem. This method allows the model to gain a deeper understanding of the underlying neurological activities. Pre-trained models can be potentially used for various downstream tasks such as sleep stage classification and gesture recognition. Unlike contrastive-based methods, which strongly rely on carefully hand-crafted augmentations and siamese structure, our approach works reasonably well with a simple transformer encoder with no augmentation requirements.
Learning neurological representations that are generic for downstream tasks in a self-supervised manner is a promising research direction for the next generation of BCI with the rise of the concept of the metaverse. We hope that our exploration of prediction targets in terms of self- supervised masked autoencoding neurological representation provides insights to the community.
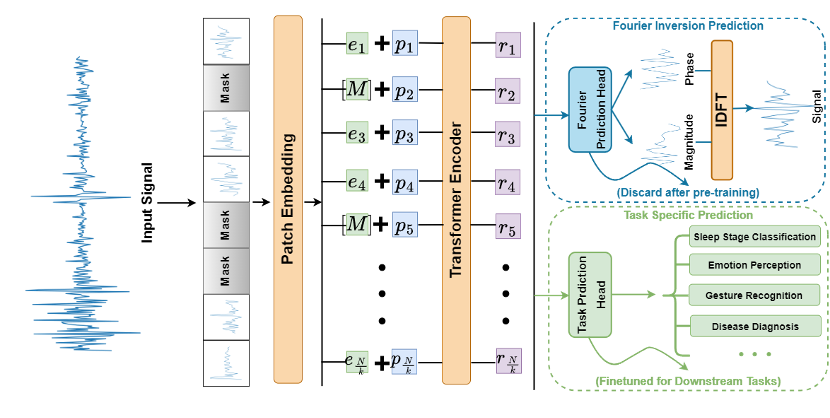
Fig.1. Illustration of the proposed Neuro-BERT for neurological self-supervised pre-training.
Research Highlights
1. We modify existing self-supervised pre-training approaches proposed for CV and NLP to accommodate neurological signals and establish them as strong baselines to alleviate reliance on massive data annotation.
2. We propose a tailored pre-training task, FIP, which predicts the missing information via the Fourier inversion theorem. We further present Neuro-BERT, a novel self-supervised pre-training framework specifically designed for neurological signals.
3. We also demonstrate that models pre-trained with FIP better capture the underlying neurological activities than naive imputation in the spatiotemporal domain.
4. Comprehensive experiments on both EEG and EMG benchmarks show that our proposed pre-training framework achieves new state-of-the-art performances.
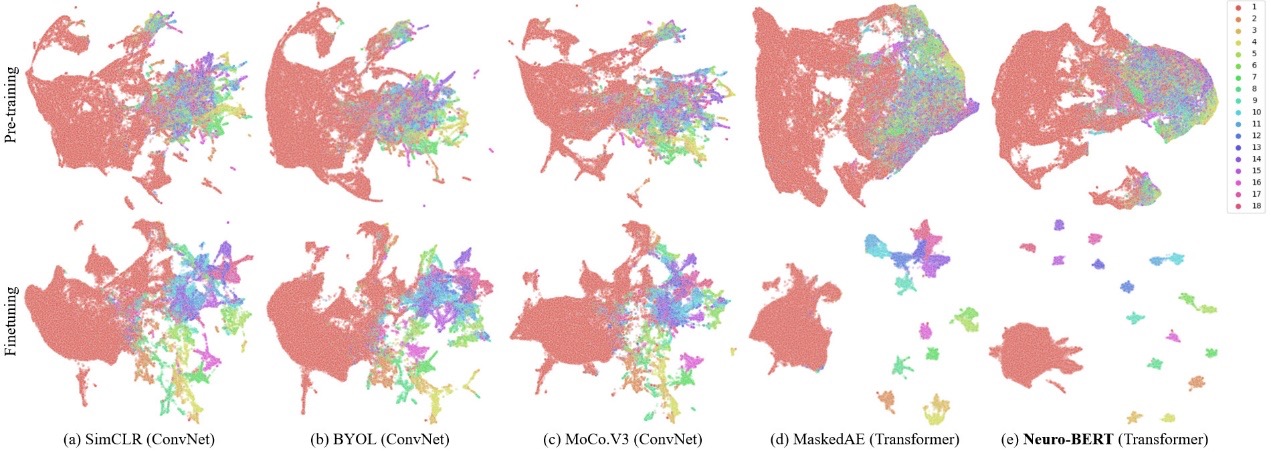
Fig.2. Visualization of pre-trained and fine-tuned embeddings with UMAP of hand gesture classification task on the Ninapro dataset.