On October 24th, 2024, the IEEE International Conference on Biomedical Circuits and Systems (BioCAS) began in Xi'an, China, marking a milestone with its 20th anniversary! Mohamad Sawan, Founding Director of CenBRAIN Neurotech and Chair Professor at Westlake University, joined two faculty members, Dr. Jie Yang and Dr. Yun-Hsuan Chen, as well as four Ph.D. students from our center in celebrating this prestigious event.
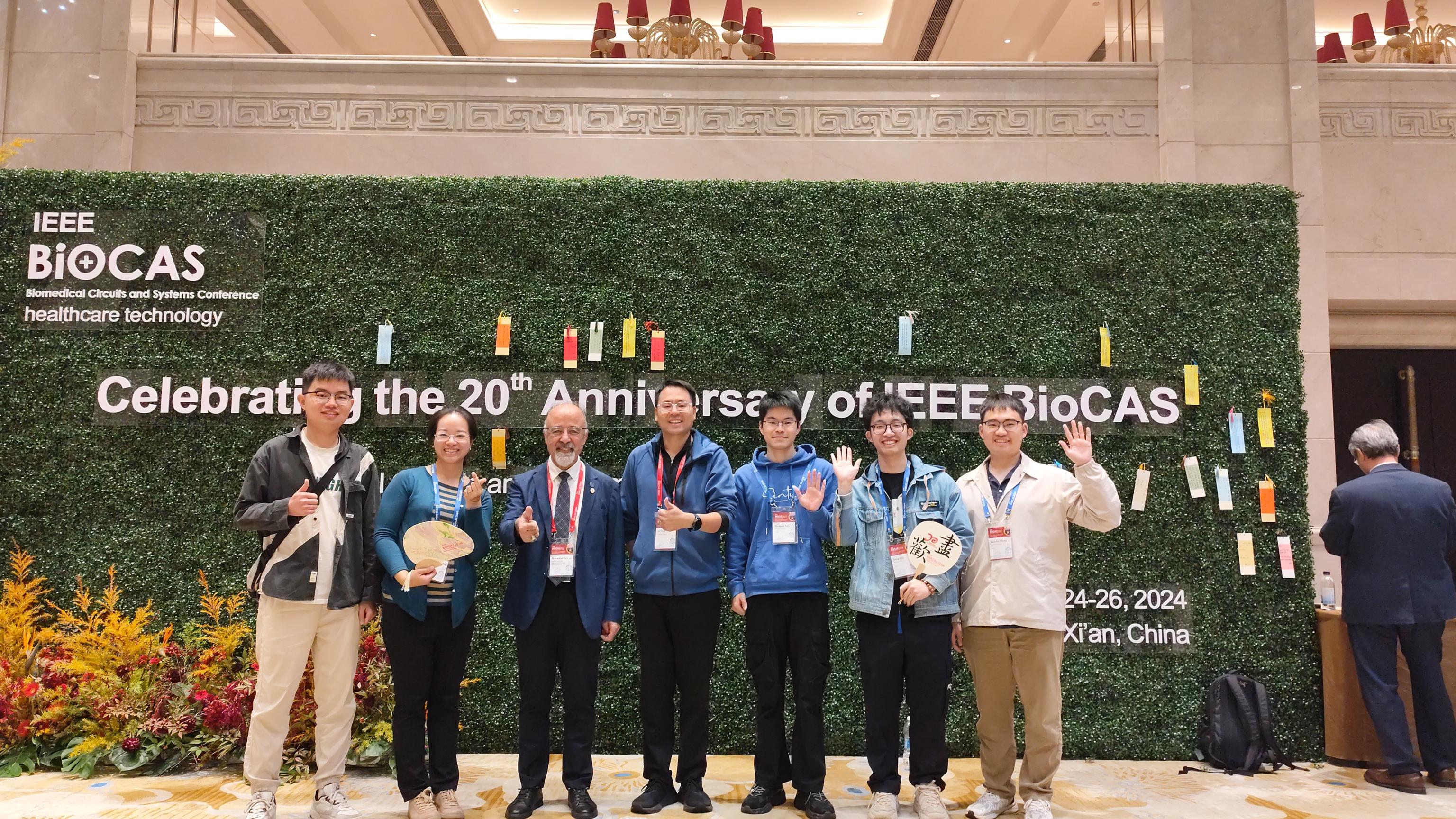
As a Life Member of IEEE and Co-chair of the BioCAS'24 20th Anniversary Planning Committee, Prof. Sawan delivered a thought-provoking keynote titled “Closed-loop Neuromodulation to Manage Brain Disorders: Treatment and Prediction.” His talk shed light on the transformative potential of brain-machine interfaces and explored current trends and challenges in the diagnosis and prognosis of brain diseases.
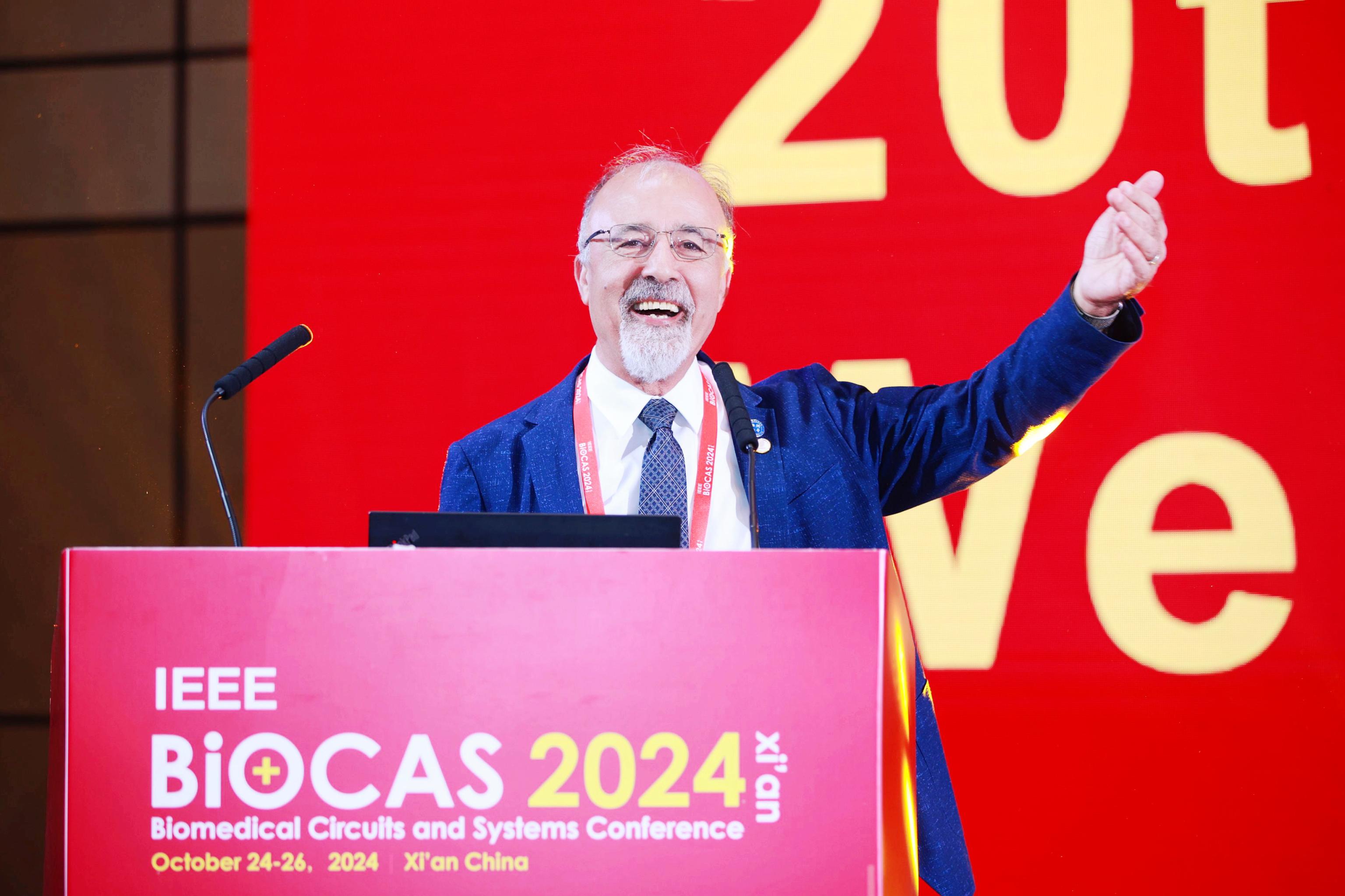
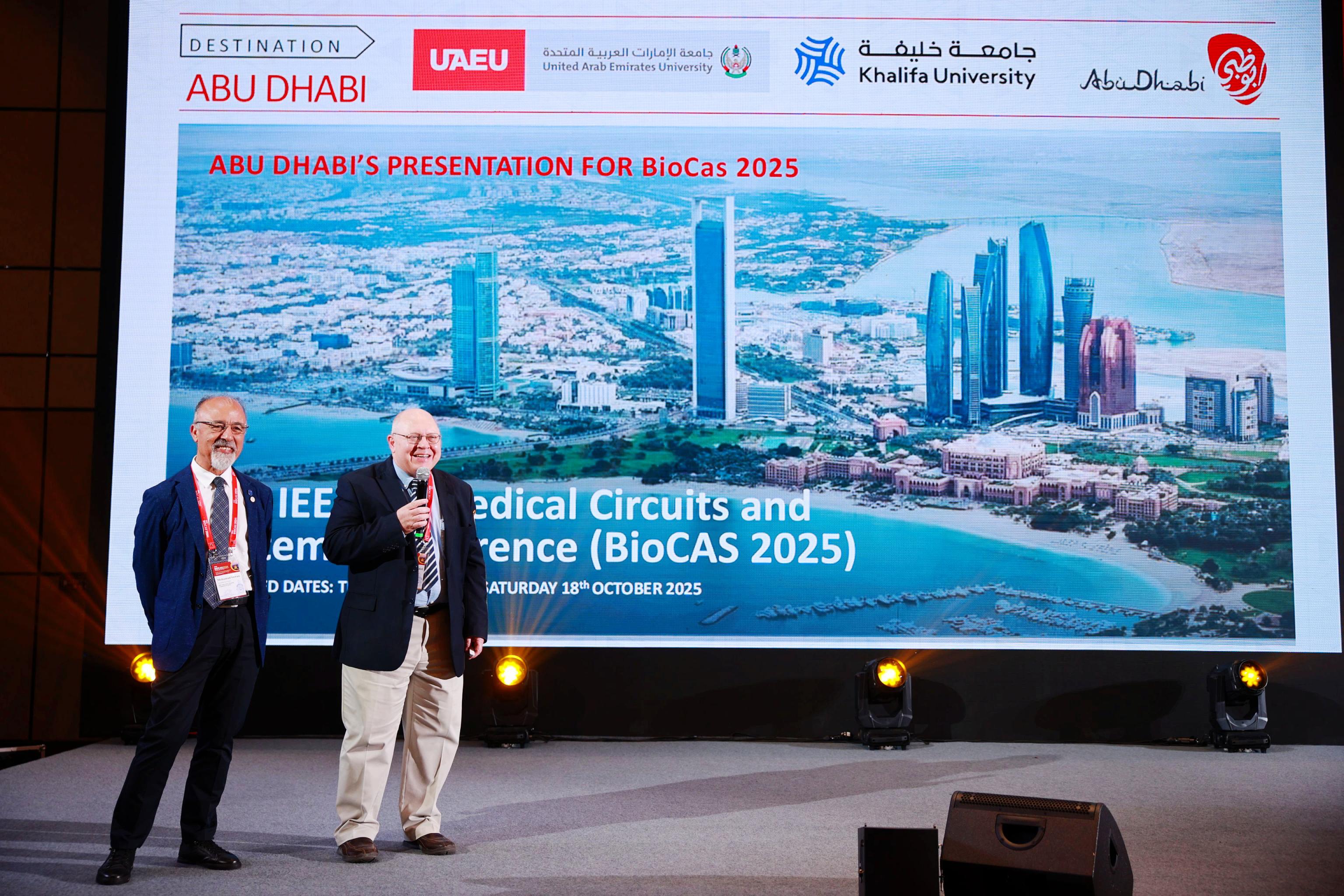
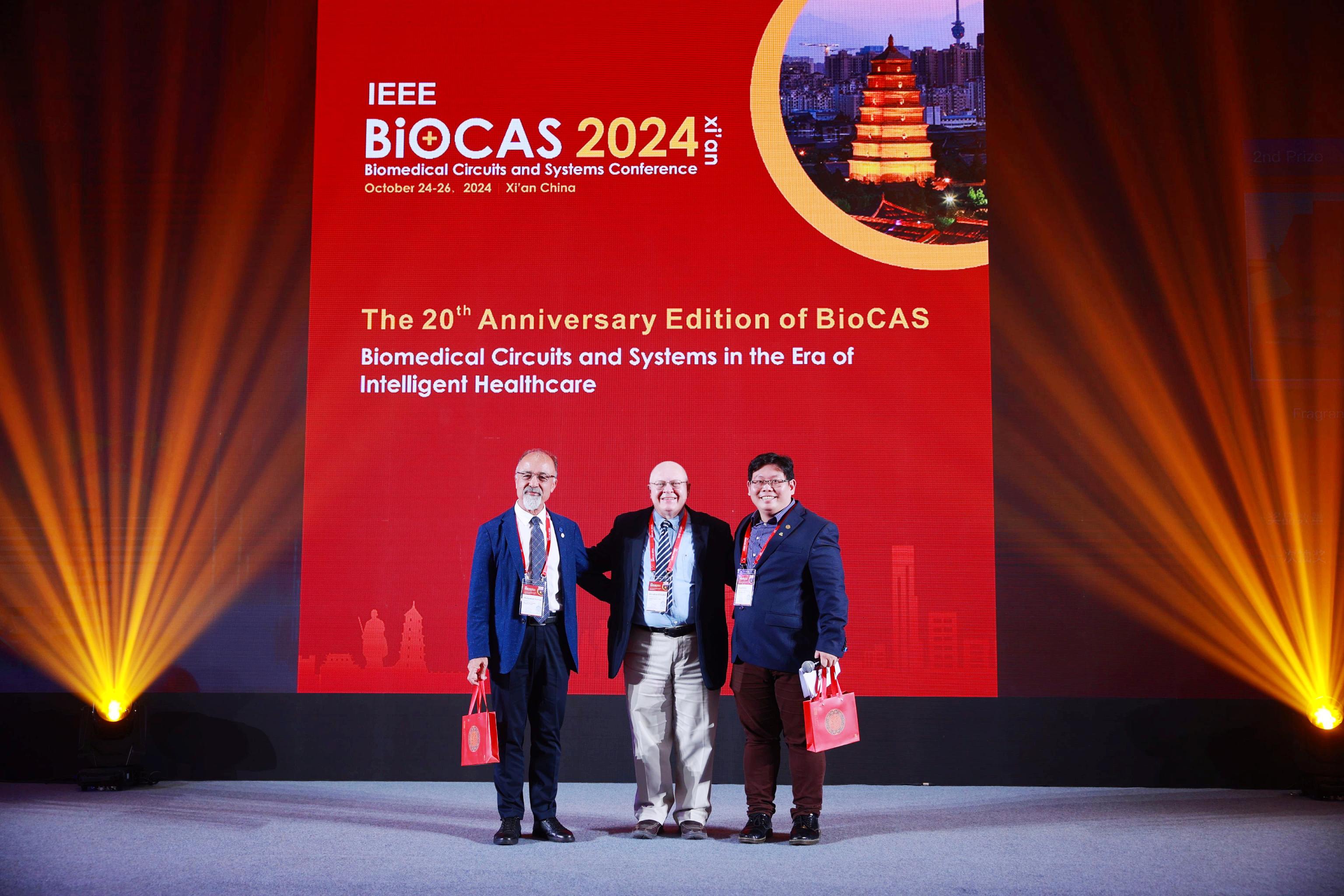
A highlight of the event was the recognition of Prof. Sawan as one of the "Top Contributors in 20 Years". Prof. Peter Lian, General Co-Chair of BioCAS’24, presented a recognition award as a tribute to Prof. Sawan’s significant academic contributions since he joined the committee in 2004 as co-founder.
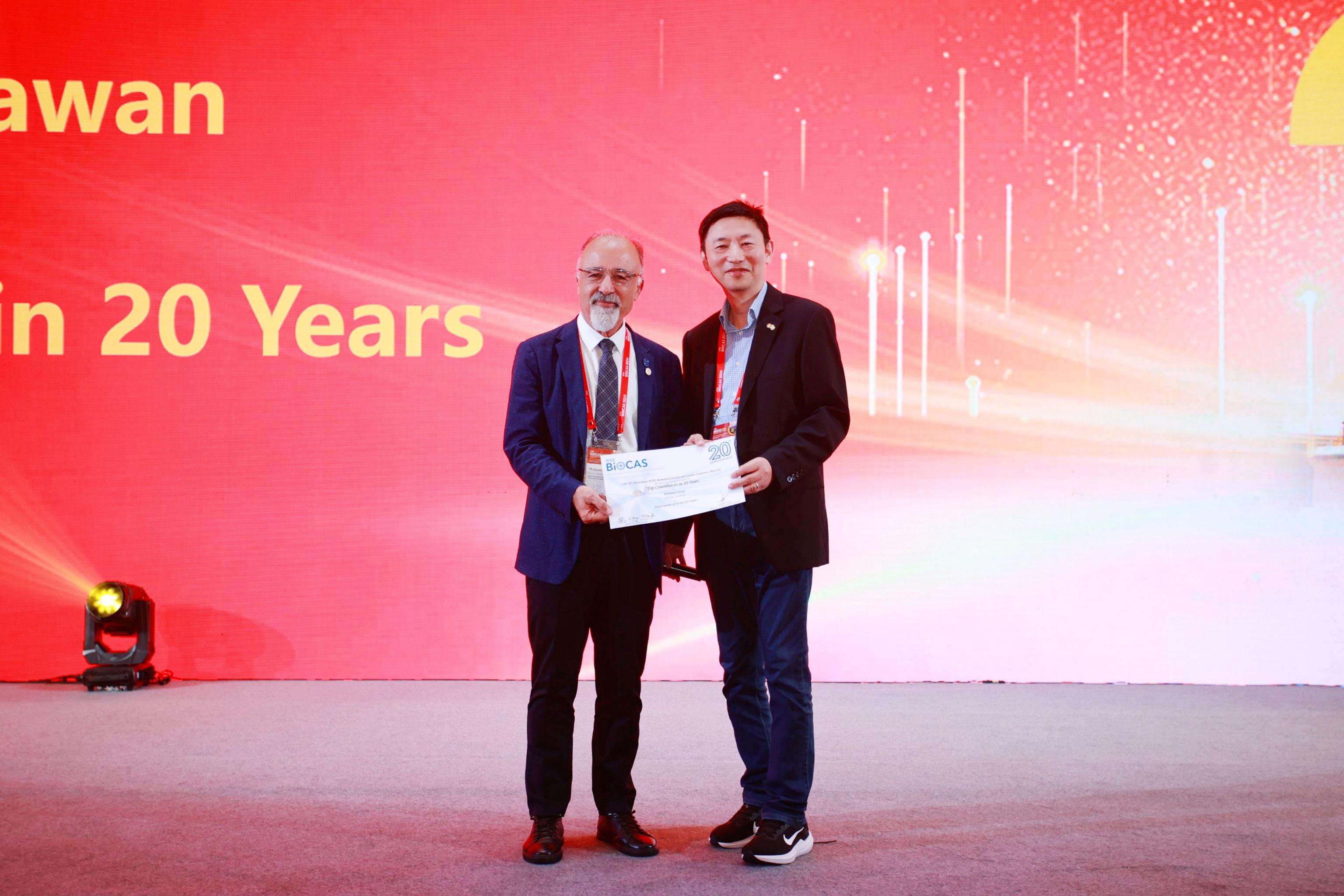
This year, our center’s four research projects were published at the conference. Ph.D. students Wenjun Zou, Xing Liu, and Junzhe Wang were invited to present their posters and the live demonstration. Additionally, a paper by our former member Wenyao Zheng was selected for an oral presentation, with Dr. Yun-Hsuan Chen, Research Assistant Professor at Westlake University, presenting in her place.
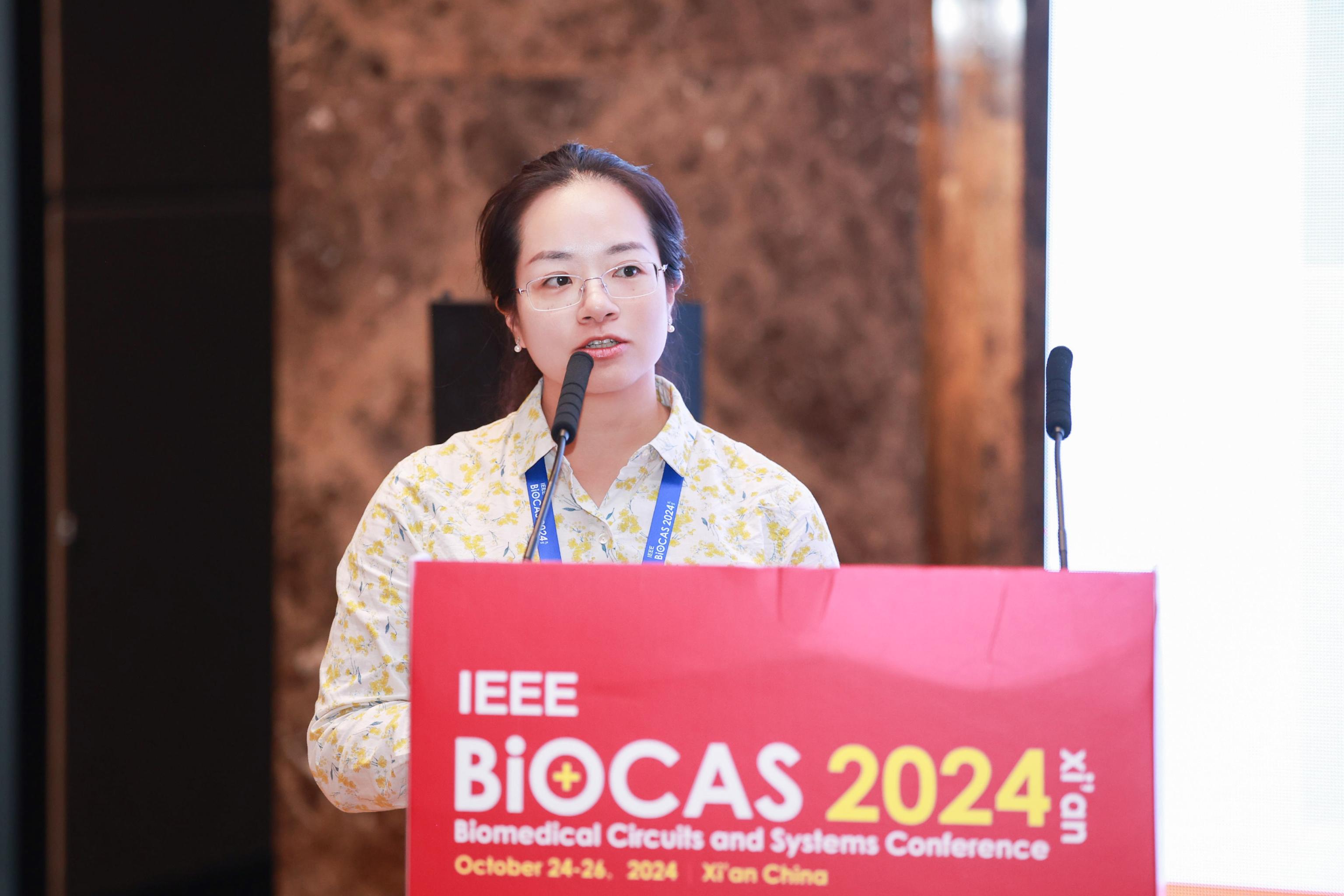
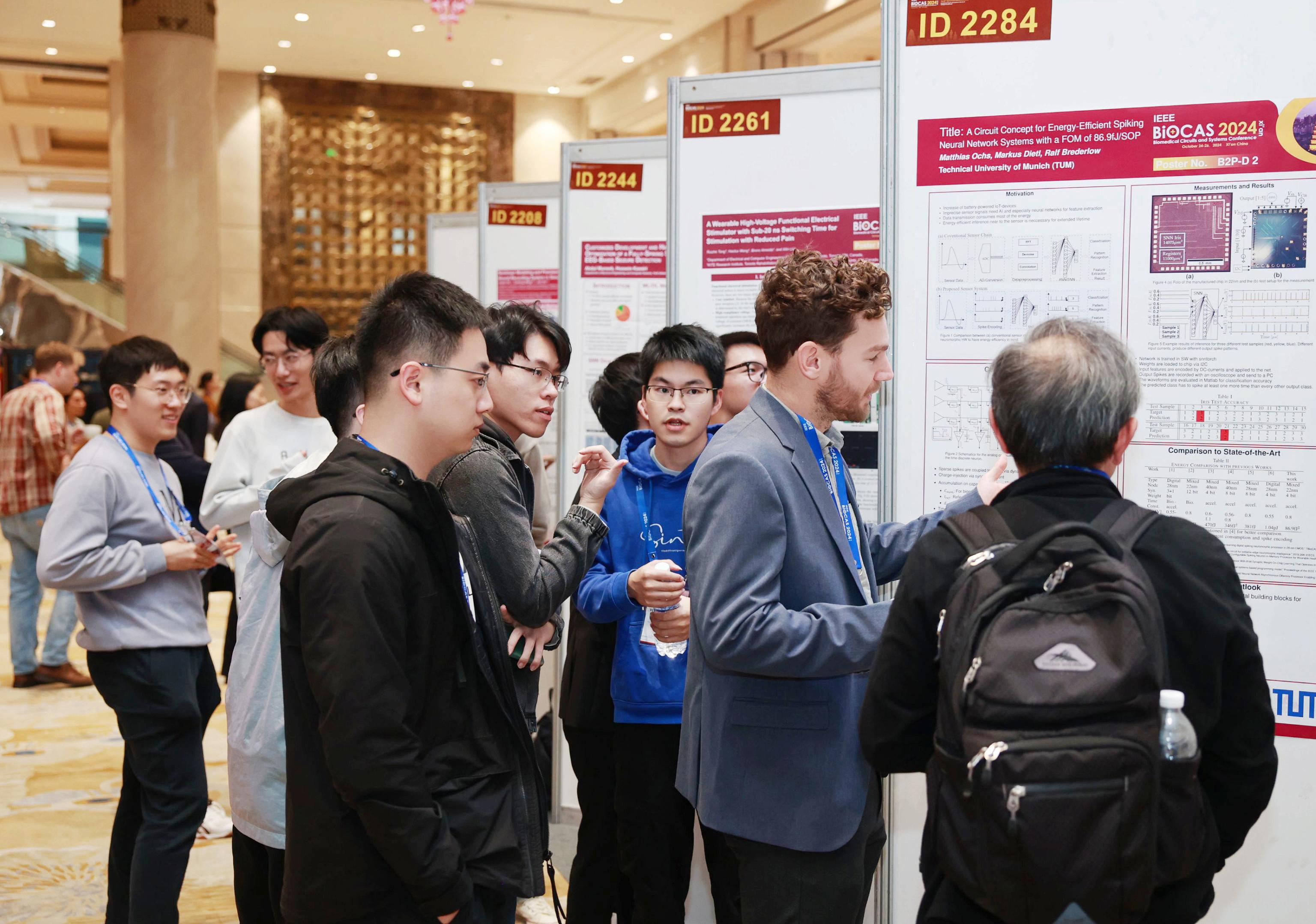
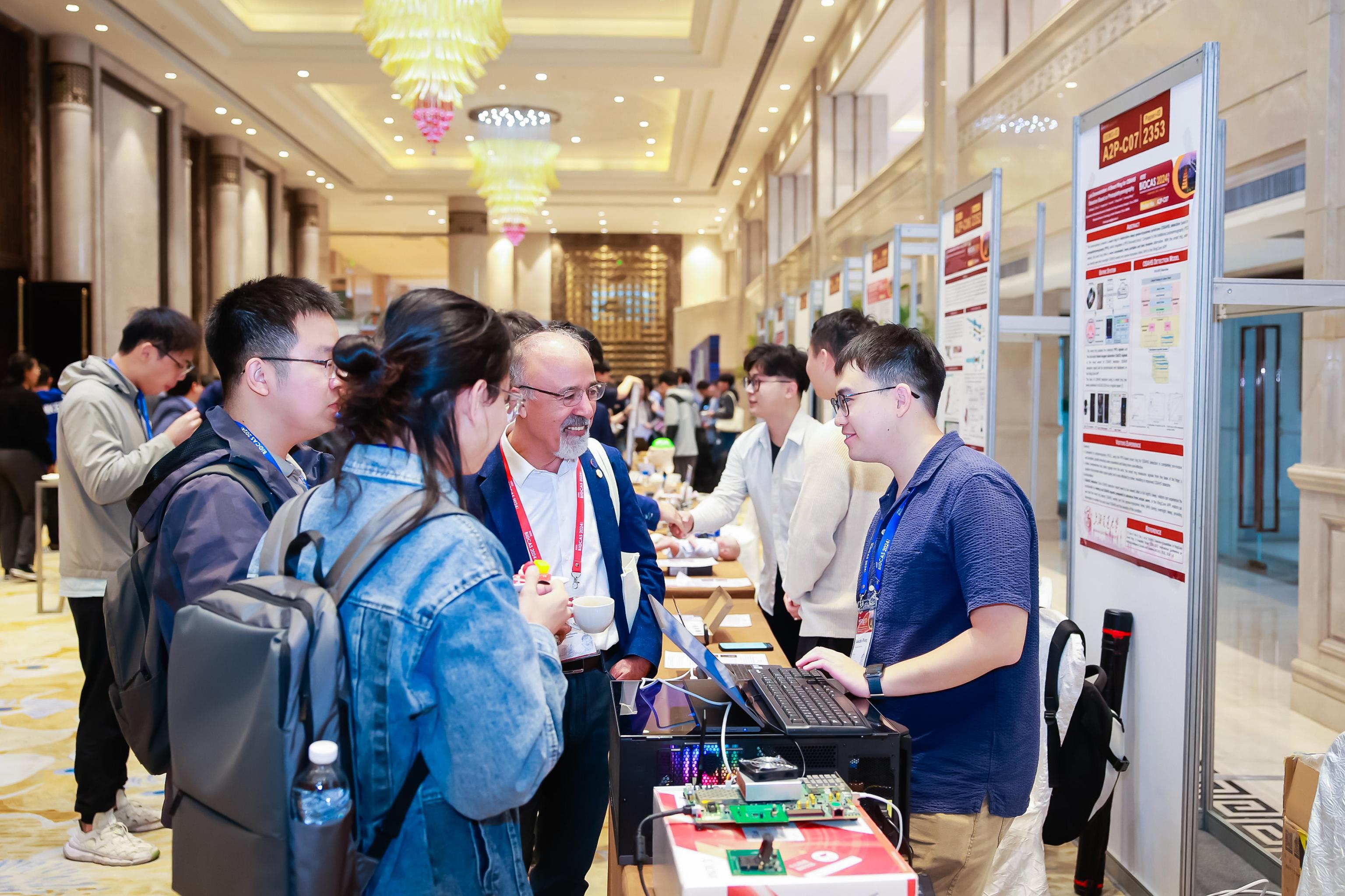
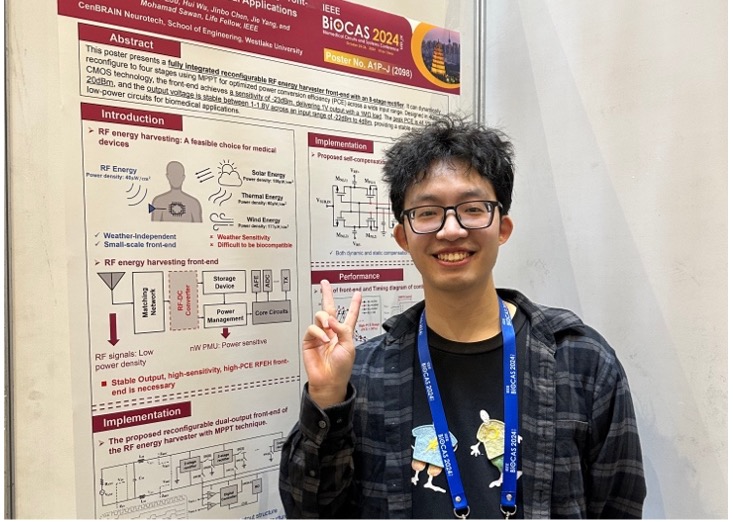
Paper#1
W. Zou, R. Eskandari, X. Liu, J. Chen, Y. Ye, H. Wu, J. Yang, M. Sawan, “A 34 μW and 3.4 pJ/B IR-UWB Transmitter Featuring Spectrum Tunability for Brain-Machine Interface,” in 2024 IEEE Biomedical Circuits and Systems Conference (BioCAS), Xi’an, China, 2024.
Abstract
We present in this paper an impulse radio ultra-wideband (IR-UWB) transmitter intended for ultra-low-power,compact, short-range, and energy-efficient applications, suchas brain-machine interfaces (BMIs). The proposed transmitterachieves effective spectrum tunability, including adjustments tocenter frequency and -10 dB bandwidth. Implemented in TSMC40 nm CMOS process technology, the chip area is 0.185 mm2, andthe post-layout simulation reveals an average power consumptionof merely 34 µW, providing an energy efficiency of 3.4 pJ/b fora 10 Mbps data transmission stream. Additionally, the peak-to-peak voltage amplitude of the UWB RF signal on a 50 Ω outputload is 450 mV.
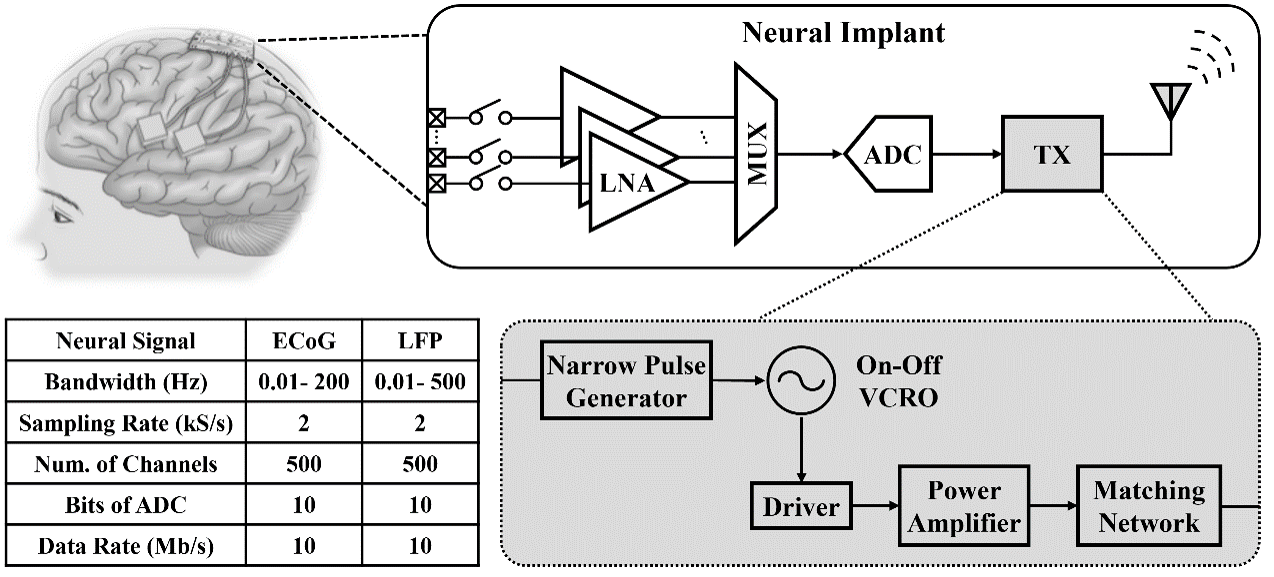
Fig. 1. Block diagram of a wireless BMI identifying the proposed IR-UWB transmitter.
图1:无线脑机接口(BMI)系统的框图和提出的IR-UWB发射器。
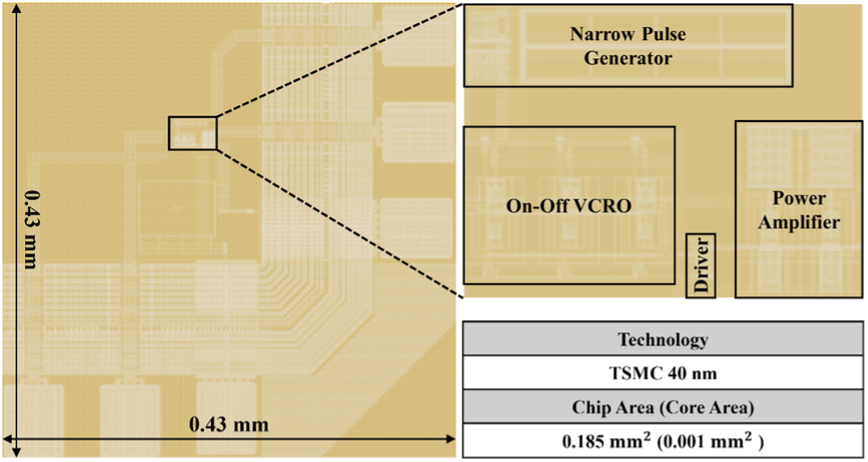
Fig. 2. Layout of the proposed IR-UWB transmitter.
图2:提出的IR-UWB发射机芯片的版图。
Paper#2
J. Wang, C. Fang, Z. Shen, J. Yang and M. Sawan, "Live Demonstration: Low-energy Unstructured Sparsity-aware Spiking Attention and Convolution SNN Accelerator," in 2024 IEEE Biomedical Circuits and Systems Conference (BioCAS), Xi’an, China, 2024.
Abstract
This live demonstration will showcase the energy efficiency and performance that a neuromorphic processor can provide for mainstream applications, including image recognition, lane and object detection in autonomous driving, and more. Visitors will have opportunities to interact with our setup and observe the real-time processing capabilities of the neuromorphic processor that was designed by CenBRAIN Neurotech. As visitors engage with the demonstration, they will see firsthand how data is transferred and processed efficiently using our proposed neuromorphic architecture. The use of offline datasets, such as ImageNet and complex traffic scene allows for a clear and simplified presentation of these capabilities. Additionally, we will display real-time measurements of power consumption, highlighting the processor’s remarkable energy efficiency.
Paper#3
X. Liu, W. Zou, H. Wu, J. Chen, J. Yang and M. Sawan, A Reconfigurable RF Energy Harvesting Front-End Interface for Biomedical Applications. in 2024 IEEE Biomedical Circuits and Systems Conference (BioCAS), Xi’an, China, 2024.
Abstract
We present in this paper a fully integrated reconfigurable front-end of RF energy harvester. The proposed 8-stage rectifier can be reconfigured into four stages with Maximum power peak tracking (MPPT) technique, enabling a wide input range of power conversion efficiency (PCE). Based on acquired radio-frequency (RF) power, the proposed front-end can be autonomously reconfigured to provide stable supply voltage for subsequent ultra-low-power circuits. Designed, implemented and simulated in standard 40nm CMOS process technology, the proposed reconfigurable front-end shows a sensitivity of -23dBm for a 1V output with a 1M$\Omega$ resistor load. The peak PCE is 46.5\% at -20dBm with 1M$\Omega$ load. Within an input power range of -22dBm to 4dBm, the output voltage of main power supply path can be maintained between 1 and 1.8V.
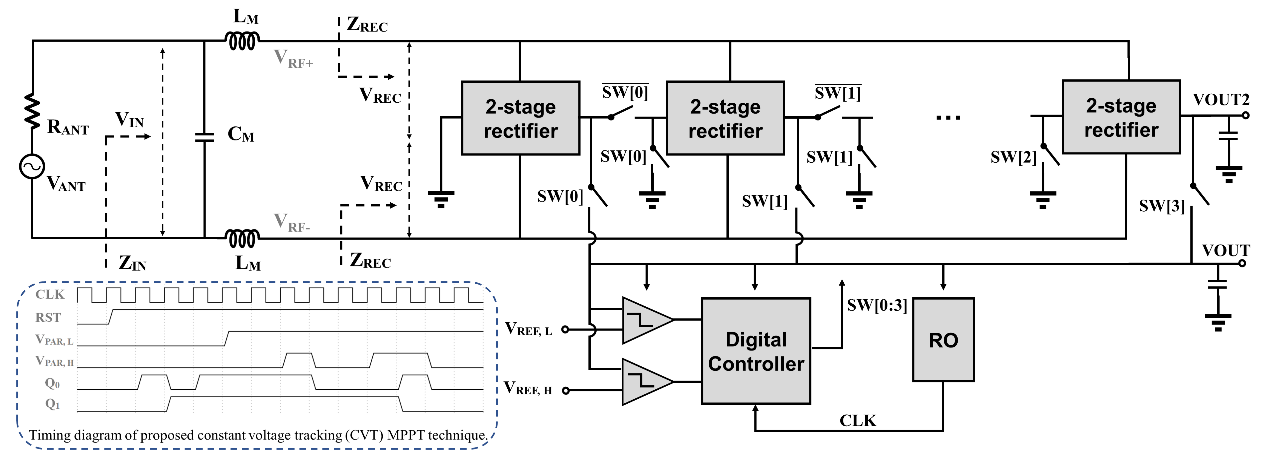
Fig.1 The proposed reconfigurable front-end of the RF energy harvester with MPPT technique.
图1:我们提出的带有最大功率点跟踪的可重构RF能量采集前端。
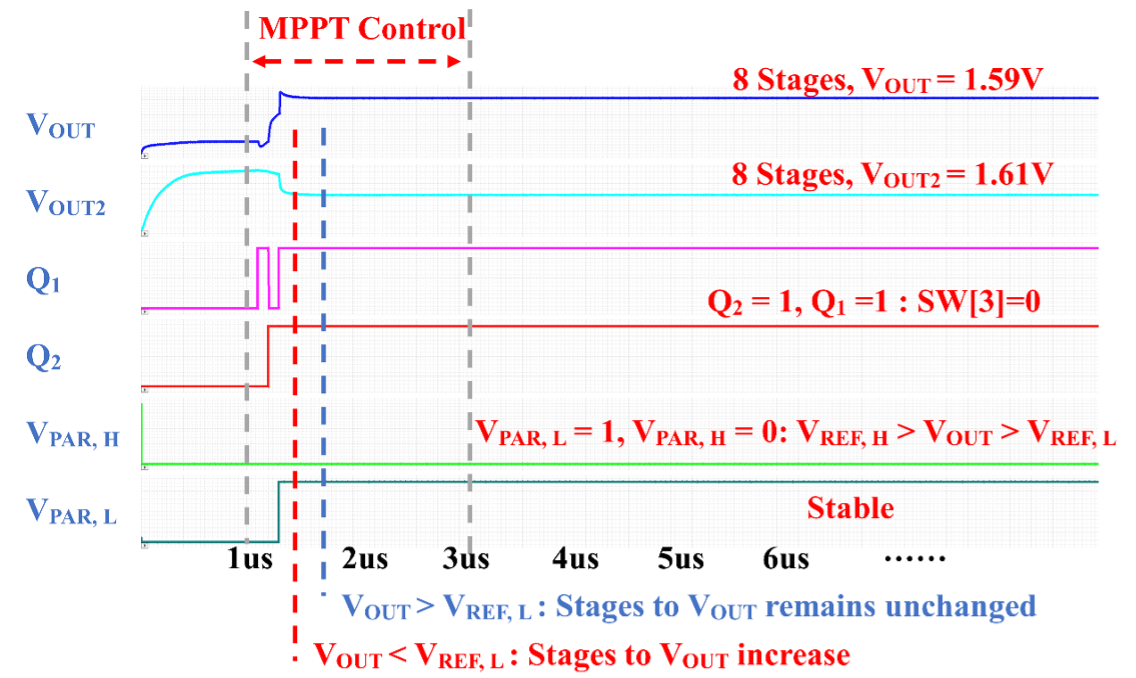
Fig.2 Timing diagram of the proposed self-compensation reconfigurable front-end with MPPT controller.
图2: 我们提出的带MPPT控制器的自补偿可重构前端的时序图。
Paper#4
W. Zheng, Y. Chen, J. WangandM. Sawan, fNIRS-based Monitoring of Functional Brain Network during Image Guided Hand Movements. In 2024 IEEE Biomedical Circuits and Systems Conference (BioCAS), Xi’an, China, 2024.
Abstract
This study investigated the effects of image guidance on uni- and bi-manual movements using functional near-infrared spec-troscopy (fNIRS), a pivotal wearable system for optical neuroimaging. We analyzed fNIRS signals from seven partici-pants performing three motor tasks: left finger tapping, right finger tapping, and finger crossing under three conditions: mo-tor execution, motor imagery, and guided motor imagery (GMI). Moreover, the motor and prefrontal cortices' functional connectivity, and brain networks were compared. Obtained results demonstrated that bimanual movements elicit tighter functional connections than unimanual movements. Additionally, image guidance made motor imagery more similar to actual movements under various features. Furthermore, the achieved results are the highest classification accuracy of 0.896 from GMI among the three motor conditions using a hybrid strategy of 5-channel statistical and 9-channel functional brain network features. Also, the outcome of this study provides insights into the functional connectivity and brain networks involved in various motor tasks, with implications for rehabilitation training and brain-machine interface applications.
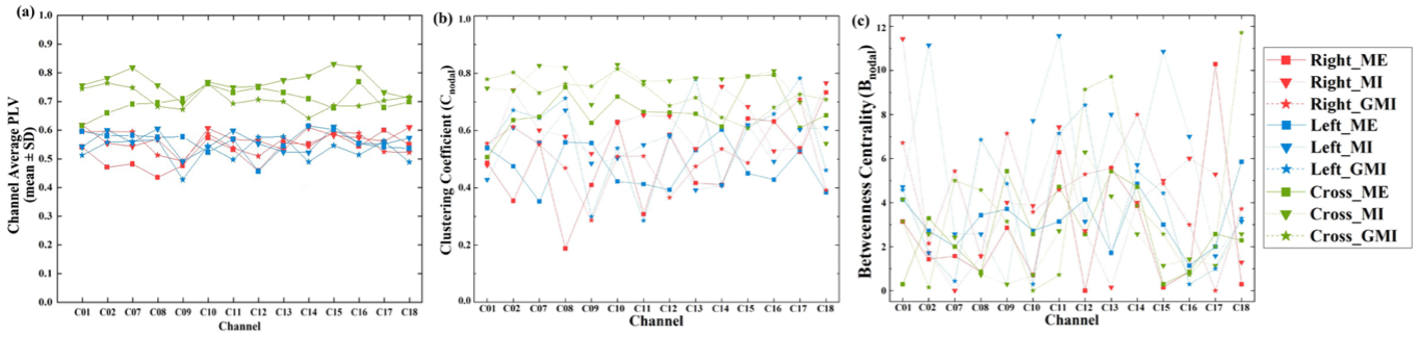
Fig.1.Results of PLV and local properties of functional brain networks using 0.69 threshold. (a) Channel average PLV under three motor conditions; (b) Clustering coefficient; (c) Betweenness Centrality.
图1:基于0.69阈值的锁相值(PLV)功能性脑网络局部特征的结果。(a)三种运动条件下的通道平均PLV;(b)聚类系数;(c)中介中心性。
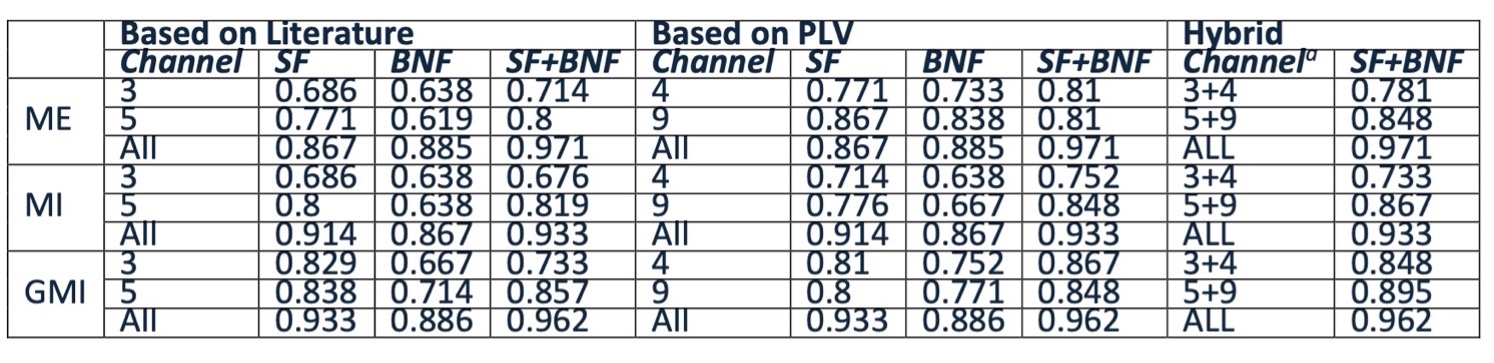
Fig.2. CLASSIFICATION RESULTS FOR THREE MOTOR TASKS UNDER ME, MI, AND GMI.
图2:基于不同特征在实际运动(ME)、运动想象(MI)、和引导运动想象(GMI)条件下三种运动任务的分类结果。
We’re proud of CenBRAIN Neurotech’s achievements! Being part of this journey in biomedical innovation drives us to keep advancing. We look forward to new breakthroughs and collaborations at BioCAS’25 in Abu Dhabi, UAE!