A recent paper from Chair Professor Mohamad Sawan's research team at Westlake University, titled Towards Homogeneous Lexical Tone Decoding from Heterogeneous Intracranial Recordings, has been successfully accepted by the International Conference on Learning Representations (ICLR). This marks the second consecutive year our research team has achieved breakthroughs at this prestigious conference.
Di Wu, a Ph.D. student from CenBRAIN Neurotech, is the first author of this work, Chair Professor Mohamad Sawan and Research Associate Professor Jie Yang is the corresponding author. The research explores the critical role of tone decoding in language comprehension and reconstruction, paving the way for advances in brain-computer interfaces.
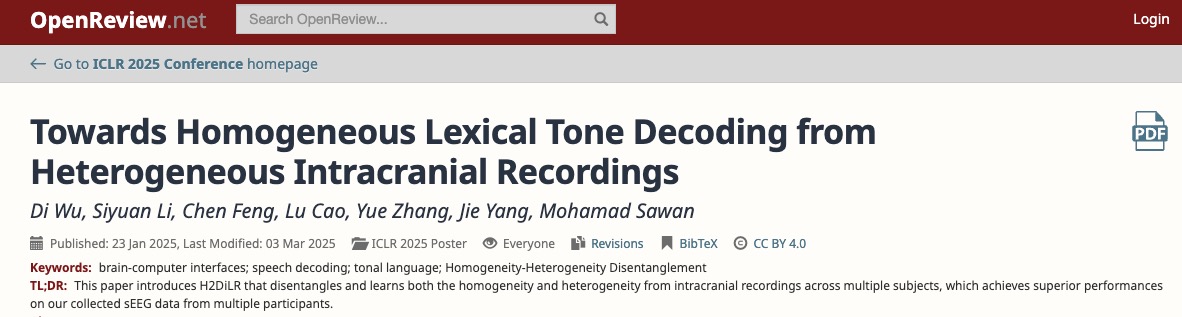
The human brain's language system is remarkably complex and sophisticated, enabling rich semantic expression. Research on decoding language from brain signals has become a popular topic in neuroscience, particularly tonal decoding, as tone plays a crucial role in over 60% of the world's languages, affecting approximately one-third of the global population. For example, in Mandarin Chinese, tone distinguishes word meanings— the same syllable "ba" can mean "eight" (八), "pull" (拔), "handle" (把), or "dad" (爸) depending on its tone. Accurate tonal decoding is essential for understanding and reconstructing tonal languages.
Abstract
This paper proposes a two-stage framework for neural decoding that enables unified multi-subject decoding by disentangling and learning both homogeneous and heterogeneous neural representations across individuals. In the first stage, Homogeneity-Heterogeneity Decoupling (H2D), vector quantization is used to extract neural features, constructing two optimizable codebooks: a shared codebook to capture commonalities across subjects (homogeneity) and multiple private codebooks to model individual-specific characteristics (heterogeneity). In the second stage, the decoding stage, the disentangled neural representations are used for efficient tonal decoding, effectively handling both shared and individual differences among subjects.
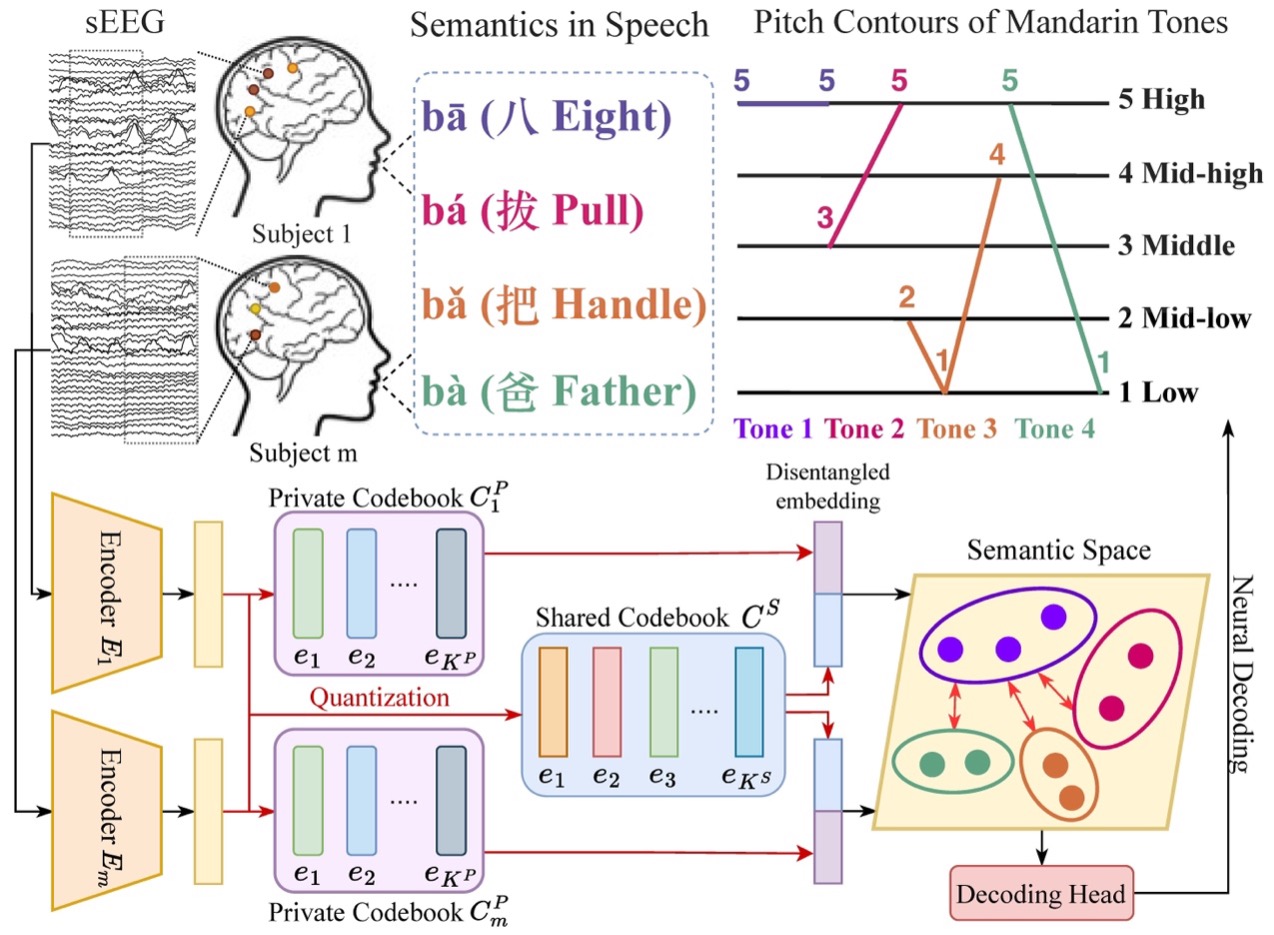
Figure1: Illustration of H2DiLR for unified lexical tone decoding with sEEG from multiple participants. In the homoheterogeneity disentanglement (H2D) stage, the continuous latent representations from the encoders are disentangled into H2D representations, which are constructed by discretized code embeddings in a shared codebook (homogeneous tone articulation neural codes) and private code books (heterogeneous personalized neural codes). The learned H2D representations are utilized for tone decoding in the second stage.
The International Conference on Learning Representations (ICLR) is internationally recognized as one of the premier conferences in the field of deep learning. It is globally renowned for presenting and publishing cutting-edge research on all aspects of deep learning used in the fields of artificial intelligence, statistics, and data science, as well as important application areas such as machine vision, computational biology, speech recognition, text understanding, gaming, and robotics.